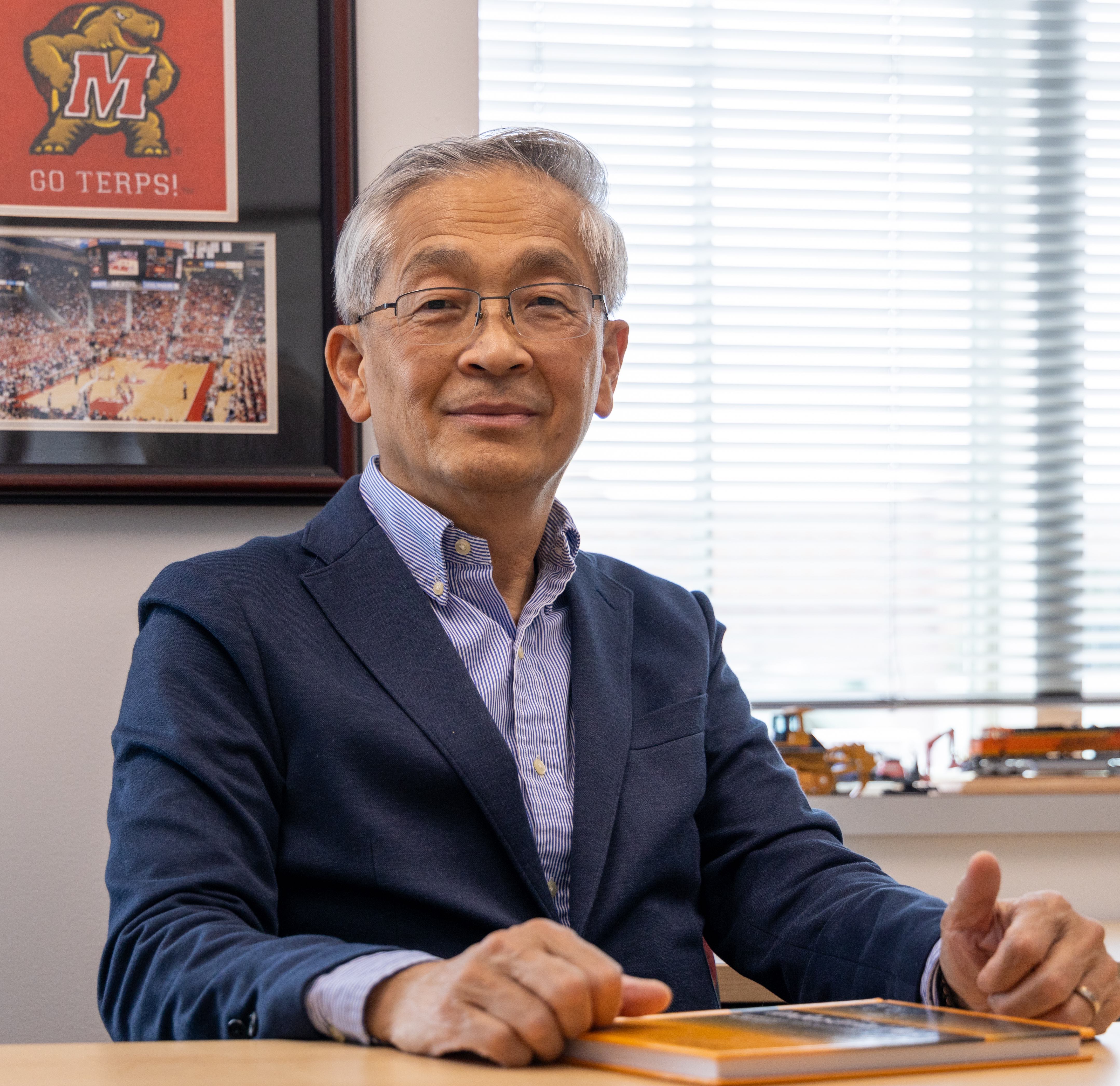
Lee, Jay
Director of Industrial Artificial Intelligence Center
Mechanical Engineering
Maryland Robotics Center
Center for Risk and Reliability
Dr. Jay Lee is Clark Distinguished Chair Professor and Director of Industrial AI Center in the Mechanical Engineering Dept. of the Univ. of Maryland College Park. His current research is focused on developing non-traditional machine learning including transfer learning, domain adaptation, similarity-based machine learning, stream-of-x machine learning, as well as industrial large knowledge model (ILKM), etc. In addition, he is leading Data Foundry which consists of over 100 diversified industrial datasets including semiconductor manufacturing, jet engines, wind turbine, EVs, high speed train, machine tools, robots, medical TBI, etc. for industrial AI talent development. These datasets are also used to rapidly develop and validate Industrial AI system with scalable and systematic approaches.
Previously, he served as an Ohio Eminent Scholar, L.W. Scott Alter Chair and Univ. Distinguished Professor at Univ. of Cincinnati. He was Founding Director of National Science Foundation (NSF) Industry/University Cooperative Research Center (I/UCRC) on Intelligent Maintenance Systems (www.imscenter.net) during 2001-2019. IMS Center has developed research memberships with over 100 global company since 2000 and was selected as the most economically impactful I/UCRC in the NSF Economic Impact Study Report in 2012. He mentored his students and developed a number of start-up companies including Predictronics through NSF iCorps in 2013. He has developed Dominant Innovation® methodology for product and service innovation design.
He was on leave from UC to serve as Vice Chairman and Board Member for Foxconn Technology Group during 2019-2021 to lead the development of Foxconn Wisconsin Science Park in Mt. Pleasant, WI (www.foxconnwiofficial.com). In addition, he advised Foxconn business units to successfully receive six World Economic Forum (WEF) Lighthouse Factory Awards since 2019.
He is a member of Global Future Council on Advanced Manufacturing and Production of the World Economics Council (WEF), a member of Board of Governors of the Manufacturing Executive Leadership Council of National Association of Manufacturers (NAM), Board of Trustees of MTConnect, as well as a senior advisor to McKinsey. Previously, he served as Director for Product Development and Manufacturing at United Technologies Research Center (now Raytheon Technologies Research Center) during 1998-2000, as well as Program Director for a number of programs (including ERCs, I/UCRCs, and Materials Processing and Manufacturing Programs) at NSF during 1991-1998.
He was selected as 30 Visionaries in Smart Manufacturing in by SME in Jan. 2016 and 20 most influential professors in Smart Manufacturing in June 2020, and has received SME Eli Whitney Productivity Award and SME/NAMRC S.M. Wu Research Implementation Award in 2022. His new book on Industrial AI was published by Springer in 2020. He is also a working group member for the recent Report on AI Engineering by NSF Engineering Research Visionary Alliance (ERVA) in 2024.
For publication citations, highlights, and recent news:
Google Scholar https://scholar.google.com/citations?user=g9GtqgQAAAAJ&hl=en&oi=ao
ResearchID: https://researchid.co/jay.lee
ResearchGate https://www.researchgate.net/profile/Jay_Lee10
ScholarGPS: https://scholargps.com/scholars/25430747289210/jay-lee
World Best Mechanical and Aerospace Professors (https://research.com/u/jay-lee ) https://research.com/scientists-rankings/mechanical-and-aerospace-engineering
Top 2% of World Scientists by Stanford Univ. 2020-2024 (overall ranking 9649 among 1,688,236, and ranked 75h out of 113,906 in the field of Industrial Engineering and Automation).https://top2percentscientists.com/stanford-elsevier-top-scientists-list-...
Editor-in-Chief, Machine Learning: Engineering, IOP Science, https://iopscience.iop.org/journal/3049-4761
AI Engineering Report, NSF ERVA Report, 2024. https://www.ervacommunity.org/visioning-report/report-ai-engineering/
WEF Davos 2023 Featured video on AI for Smart Manufacturing. https://app.frame.io/presentations/c6b734dd-9db2-4900-bab2-903ace86979f
WEF interview article https://theinnovator.news/interview-of-the-week-jay-lee-industrial-ai-expert/
Industrial AI, Industrial Big Data, Prognostics and Health Management (PHM), Smart Manufacturing, Digital Twin, etc.
1. Intelligent Metrology Systems of Advanced Semiconductor Manufacturing.
2. Smart Electrification Analytics for EV systems.
3. AI Augmented ICU and Medical Analytics (TBI)
4. Cyber Phyiscal Systems and Digital Twin for Smart Manufacturing.
5. Prognostics of Highly Connected and Complex Systems including Wind Turbine/Wind Farm, High Speed Train, Aircraft Fleet, Factoty Automation and Robot Lines, Semiconductor Fab., etc.
6. Intelligent Maintenance Systems for Mechanical Components (bearing, ball screw, pump, valve, motors, etc) and sensors/sensory systems.
Partial List of Current Research Projects:
Winbond Electronics Corporation |
Winbond Electrostatic (ESC) Chuck Remaining Useful Life |
Hitachi High-Technologies Corporation |
Phase V: Chamber Matching based on Etching Digital Twin Model |
Applied Materials, Inc. |
Multivariate Simulation Dataset Generation for Fault Detection with Semi-Automated Feature Extraction and Semi-supervised Limits Setting Applied to Semiconductor Manufacturing Processes |
Mitsubishi Electric Corporation |
Health Assessment & Fault Detection for Industrial Robots |
Hitachi High-Technologies Corporation |
Phase 2021-1: Development of digital twin model for Hitachi plasma etching tool and calibration of tool performance shift |
United Microelectronics Corporation (UMC) |
Applications of Machine Learning Operation Techniques |
National Institute of Standards and Technology |
Industrial Artificial Intelligence Consortium to Advance High-Mix Production Systems |
MxD |
Predictive Maintenance Analytics of Roll-to-Rolll Manufacturing |
|
|
|
|
|
|
|
|
|
|
|
|
|
|
|
|
|
|
Industrial AI (Course Number ENME 691 or ENME 485)
Term: Fall/2023
Professor: Jay Lee
Pronouns: He/His/Him
Office Phone: 301-405-5205
Email: leejay@umd.edu
Office Hours: Monday 9 am- 11am, Jeong H. Kim Engineering Building 3226
Teaching Assistant: Dai-Yan Ji, Takanobu Minami
Pronouns: He/His/Him
Email: jidn@umd.edu & minamitu@umd.edu
Office Hours: Tuesday&Thursday 2pm- 3pm, Jeong H. Kim Engineering Building 1210
Credits: 3
Course Dates: From August 28, 2023 - December 11, 2023
Course Times: Monday 5 pm-7:40 pm
Course Description
In today’s competitive business environments, companies have urgent needs to use advanced analytical tools to manage their industrial data to gain more insights of their operations. Those insights include machine health condition, system remaining useful life, system performance, and other key performance indicators. With the advent of networked devices, an abundance of data now exists in virtually every level of industry, from the individual manufacturing asset to the manufacturing facility as well as the entire organization. This data is often not used to its greatest potential. It is often acquired, stored and forgotten. Most organizations understand the need for acquiring data, but do not understand how to leverage the data to enhance their system design, condition monitoring and decision-making capabilities. This course will introduce students to advanced technologies—such as machine learnings and tools, prognostics and health management (PHM), data-centric engineering analytics—that ultimately enable the conversion of industrial big data into decision-ready information that can be used to improve the design, the productivity and the efficiency of industrial systems.
Learning Outcomes
After successfully completing this course you will be able to:
- Learn the fundamental issues in data quality engineering for machine learning as well as utilize intelligent AI tools for machine monitoring, performance assessment, and prognostics of complex industrial systems.
- Understand the systematic approach in developing, validating, and deploying machine learning tools in using the real-world industrial datasets.
- Apply the learned Industrial AI tools to the assigned team project through an integrated learning of domain, data, and discipline system approach.
Required Resource Course Website: elms.umd.edu There will be no formal textbook for this class, but all lecture notes will be posted online. The reference below is strongly recommended to use on a regular basis throughout the semester.
- Text Book:
1. Jay Lee, Industrial AI: Applications with Sustainable Performance, Springer, 2020.
- Reference Books:
1. Bishop, Christopher M. Pattern recognition and machine learning. springer, 2006.
2. Gelman, Andrew, et al. Bayesian data analysis. Vol. 2. London: Chapman & Hall/CRC, 2014.
3. Randall, Robert Bond. Vibration-based condition monitoring: industrial, aerospace and automotive applications. John Wiley & Sons, 2011.
- Software: MATLAB or Python
Prerequisite and Criteria for the Course
Abilities in using MATLAB or Python or Permission from the Instructor
Course Structure
This course belongs to in-person session (on-line Recording will be arranged for students to review). Students are expected to attend all classes (please be on time). Even though most of the course materials are well covered by the posted lecture notes and text, there may be some information that is discussed exclusively in class. If you missed class, you are still responsible for learning the materials taught during your absence.
Major Assignments
Homework Assignments
- Assignment#1
1. Review the assigned publications, reports, and white papers.
2. Survey the literature to gain the understanding of the role of AI in industrial 4.0, digital transformation, and smart manufacturing.
3. Each student will develop a survey report and give a 5-minute presentation to share the findings and summary with the class.
- Assignment#2
1. A bearing vibration dataset and specific homework requirements will be given to the student. The
dataset is generated from the vibration test in the IAI center.
2. Sample code for Fast Fourier Transform and logistic regression will be provided to the student to finish
the homework.
3. Students will be divided into working groups for homework.
4. Each group will give a 10-minute presentation in the class.
- Assignment#3
1. The student groups are asked to use the Support Vector Machine (SVM) and other algorithms to detect and diagnose the bearing failures. Sample code for SVM will be provided.
2. The assignment will be finished by the group, and each group is asked to report their work in the class
through a 10-minute presentation.
- Assignment#4
1. The student groups are asked to use the Self-Organizing Map (SOM) and SOM-MQE to detect and diagnose the bearing failure. Sample code for SOM and SOM-MQE will be provided to the class to finish the
homework.
2. Each group is asked to report their work in the class through a 10-minute presentation.
- Project 1: Fault detection in semiconductor Etching
Project 2: Gearbox fault diagnosis and prognosis
Project 3: Bearing fault diagnosis and prognosis.
Project 4: Virtual metrology for critical semiconductor manufacturing processes
Project 5: Machine tool degradation prognosis and remaining useful life prediction.
Project 6: Aero-engine remaining useful life prediction
Project 7: EV Batteries
Project 8: Spacecraft propulsion system
- In the final project, each student group will focus on one of the 8 projects that are listed above. The dataset and detailed requirements will be given to students to start the project. TA will be assigned to each group to provide support and answer questions. Each group will work independently to fulfill the requirements in the final project. In the exam week, each group will make a 15-minute presentation to report their work and document all the details in a report. When promising results are achieved or novel methods are proposed, the instructor will advise the student group to publish papers at conferences or research journals.
- The concept of design these projects is to motivate students to learn by address real-world problems independently. The datasets used in these final projects are from IAI past research projects or open data competitions. They are all real-world datasets generated from machines or production lines. Although each student group will focus on one of the 8 projects in this class, the datasets of all these projects will be made accessible to all the students for their future use.Grading Structure
Assignment |
Percentage % |
Homework & Presentation |
20% |
Midterm Test |
40% |
Final Term-Project Report |
40% |
Total |
100% |
Course Outline
Week # |
Topic |
Assignment |
(8/28) 1 |
Industrial AI Introduction (Part 1): Data Issues on Industrial Big Data System: Data Source, Data Quality, and Data Context |
HW1 |
(9/11) 2 |
Invited Lecture: Prof. Alex Jia, Univ. of Cincinnati Fundamentals of Signal Processing and Feature Extraction and Commonly used tools and Case studies in Industrial AI and Industrial AI (Part 2) |
|
(9/18) 3 |
Industrial AI Introduction (Part 3): Machine Learning tools (Part 1): Logistic Regression & Student HW 1 Presentations and Discussions |
HW2 (Bearing data sets) |
(9/25) 4 |
Machine Learning tools (Part 2): Support Vector Machine, Self-Organizing Map, K-Means and Others. and Student HW 2 Presentations and Discussions |
HW3 (Bearing – SVM) |
(10/2) 5 |
Review ML Tools and Case Studies Machine Health Monitoring using Industrial Big Data: Case Study I: Machine Level Heath Monitoring Case Study II: System Level Health Monitoring and Student HW 3 Presentations and Discussions |
HW4 (Bearing – SOM) |
(10/9) 6 |
Invited Speaker: David Siegel, CTO of Predictronics (TBD) Industrial AI tool for Prognostics and Health Management and Introduction to PHM Data Challenge and Case Studies and Student HW 4 Presentations and Discussions, Preview Midterm Preparation |
|
(10/16) 7 |
Midterm |
|
(10/23) 8 |
Midterm Review and Industrial AI for Networked and Complex Engineering Systems: Case Study III: Wind Turbine and Wind Farm Case Study IV: Smart EV Battery & Mobility |
|
(10/30) 9 |
Introduction of Group Projects and Assignments Project 1: Fault detection in semiconductor Etching Project 2: Gearbox fault diagnosis and prognosis Project 3: Bearing fault diagnosis and prognosis. Project 4: Virtual metrology for critical semiconductor manufacturing processes Project 5: Machine tool degradation prognosis and remaining useful life prediction. Project 6: Aero-engine remaining useful life prediction Project 7: EV Batteries Project 8: Spacecraft propulsion system |
|
(11/6) 10 |
Group Project Presentation 1 |
|
(11/13) 11 |
Invited Guest Lecture Group Project Presentation 2 |
|
(11/20)12 |
Group Project Presentation 3 |
|
(11/27)13 |
Invited Lecture: Prof. Kiritsis Dimitris, EPFL, Switzerland Group Project Presentation 4 |
|
(12/4) 14 |
Final Group Project Presentation |
|
(12/11) 15 |
Final Report Due |
|
-
Researcher ID: https://researchid.co/jay.lee
-
ResearchGate: https://www.researchgate.net/profile/Jay-Lee-27
- Lee, Jay. Industrial AI: Applications with Sustainable Performance, Springer, ISBN 978-981-15-2143-0, 2020.
Book Chapter:
- Book Chapter, Lee, J. etc. 'Cyber-Physical System Framework of AI in Manufacturing and Maintenance,” Book on “Artificial Intelligence in Manufacturing,” Elsevier, 2023.
- Book Chapter, Analyzing Data Obtained via Wind Farm Supervisory Control and Aata Acquisition,” Book on Utility-Scale Wind Turbines and Wind Farms, Institution of Engineering and Technology (IET), ISBN: 9781839530999, e-ISBN: 9781839531002, 2020.
Special Issues:
- Guest Editor, Special Issue on "Artificial Intelligence for Cyber-Enabled Industrial Systems" Journal of Machines, 2018.
- Guest Editor, Data-Driven Cognitive Manufacturing - Applications in Predictive Maintenance and Zero Defect Manufacturing, Organized by: Dimitris Kiritsis, Melinda Hodkiewicz, Oscar Lazaro, Jay Lee, Journal of Frontiers in Computer Science - Mobile and Ubiquitous Computing, 2020.
-
-
Taco, John, Pradeep Kundu, and Jay Lee. "A novel technique for multiple failure modes classification based on deep forest algorithm." Journal of Intelligent Manufacturing (2023): 1-15. [link]
-
Kundu, Pradeep, Marcella Miller, Prayag Gore, Xiaodong Jia, and Jay Lee, "Detection of inception of preload loss and remaining life prediction for ball screw considering change in dynamics due to worktable position," Mechanical Systems and Signal Processing 189 (2023): 110075.
-
Leitão, Paulo, Stamatis Karnouskos, Thomas I. Strasser, Xiaodong Jia, Jay Lee, and Armando Walter Colombo, "Alignment of the IEEE Industrial Agents Recommended Practice Standard with the Reference Architectures RAMI4. 0, IIRA, and SGAM." IEEE Open Journal of the Industrial Electronics Society 4 (2023): 98-111.
-
Hsu, Yuan-Ming, Dai-Yan Ji, Marcella Miller, Xiaodong Jia, and Jay Lee, "Intelligent Maintenance of Electric Vehicle Battery Charging Systems and Networks: Challenges and Opportunities." International Journal of Prognostics and Health Management 14, no. 3 (2023).
-
Lee, Jay, Huwei Dong, and D. J. Pradeep, "Cyber-physical systems framework for predictive metrology in semiconductor manufacturing process." International Journal of Precision Engineering and Manufacturing Smart Technology 1 (2023): 107-113.
-
Zhu, Feng, Jianshe Feng, Min Xie, Lishuai Li, Jingzhe Lei, and Jay Lee, " An Optimization-based Subset Selection and Summarization Method for Profile Data Mining," IEEE Transactions on Industrial Informatics (2023).
-
Pandhare, V., Miller, M., Vogel, W., Lee, J., Ball Screw Health Monitoring With Inertial Sensors, IEEE Transactions on Industrial Informatics, Volume: 19, Issue 6, (2023).
-
Vibhor Pandhare, Elisa Negri; Lorenzo Ragazzini; Laura Cattaneo; Marco Macchi; Jay Lee, Digital Twin-enabled robust production scheduling for equipment in degraded state, Journal of Engineering Applications of Artificial Intelligence, (2023).
-
Lin, AC, Bpharm, MS, Lee, J., Gabriel, MK, Arbet, RN, Ghawaa, Y, Fergus, AM, The Pharmacy 5.0 framework: A new paradigm to accelerate innovation for large-scale personalized pharmacy care, American Journal of Health-System Pharmacy, September 6, 2023. https://doi.org/10.1093/ajhp/zxad212
-
Feng Zhu, Xiaodong Jia, Wenzhe Li, Min Xie, Lishuai Li, Jay Lee, Cross-chamber Data Transferability Evaluation for Fault Detection and Classification in Semiconductor Manufacturing, IEEE Transactions on Semiconductor Manufacturing, 2022
-
Jay Lee, Pradeep Kundu, Integrated Cyber-Physical System and Industrial Metaverse for Remote Manufacturing, Manufacturing Letters, 2022
-
Jay Lee, Prayag Gore, Xiaodong Jia, Shahin Siahpour, Pradeep Kundu, Keyi Sun, Stream-of-Quality Methodology for Industrial Internet-Based Manufacturing System Manufacturing Letters, 2022
-
Wenzhe Li, Xiaodong Jia, Yuan-Ming Hsu, Chen-Hong Liao, Yunfei Wang, Mong-Tung Lin, Jay Lee, A Novel Methodology for Lens Matching in Compact Lens Module Assembly, IEEE Transactions on Automation Science and Engineering, 2022
-
Shahin Siahpour, Xiang Li, Jay Lee, A Novel Transfer Learning Approach in Remaining Useful Life Prediction for Incomplete Dataset, IEEE Transactions on Instrumentation and Measurement, 2022
-
Yuan-Ming Hsu, Xiaodong Jia, Wenzhe Li, Panayotis Manganaris, Jay Lee, Sequential Optimization of the Injection Molding Gate Locations Using Parallel Efficient Global Optimization, The International Journal of Advanced Manufacturing Technology, 2022
-
Jay Lee, Shahin Siahpour, Xiaodong Jia, Patrick Brown, Introduction to Resilient Manufacturing Systems, Manufacturing Letters 2022, pp. 24-27
-
Vibhor Pandhare, Marcella Miller, Greg Vogl, Jay Lee, Ball Screw Health Monitoring with Inertial Sensors, IEEE Transactions on Industrial Informatics, 2022
-
Ainapure, Abhijeet, Shahin Siahpour, Xiang Li, Faray Majid, Jay Lee, Intelligent Robust Cross-Domain Fault Diagnostic Method for Rotating Machines Using Noisy Condition Labels, Mathematics, 2022
-
Cai, Haoshu, Jianshe Feng, Qibo Yang, Fei Li, Xiang Li, Jay Lee, Reference-based Virtual Metrology Method with Uncertainty Evaluation for Material Removal Rate Prediction based on Gaussian Process Regression, The International Journal of Advanced Manufacturing Technology, 2021
-
Cai, Haoshu, Xiaodong Jia, Jianshe Feng, Qibo Yang, Wenzhe Li, Fei Li, Jay Lee, A Unified Bayesian Filtering Framework for Multi-horizon Wind Speed Prediction with Improved Accuracy, Renewable Energy, 2021
-
Jianshe Feng, Xiaodong Jia, Haoshu Cai, Feng Zhu, Xiang Li, Jay Lee, Cross Trajectory Gaussian Process Regression Model for Battery Health Prediction, Journal of Modern Power Systems and Clean Energy, 2020
-
Feng Zhu, Xiaodong Jia, Marcella Miller, Xiang Li, Fei Li, Yinglu Wang, Jay Lee. Methodology for Important Sensor Screening for Fault Detection and Classification in Semiconductor Manufacturing. IEEE Transactions on Semiconductor Manufacturing. 10 November 2020. Pages 65-73.
-
Vibhor Pandhare, Xiang Li, Marcella Miller, Xiaodong Jia, Jay Lee. Intelligent Diagnostics for Ball Screw Fault through Indirect Sensing using Deep Domain Adaptation. IEEE Transactions on Instrumentation and Measurement. Volume 70. 9 December 2020.
-
Cai, Haoshu, Jianshe Feng, Feng Zhu, Qibo Yang, Xiang Li, Jay Lee. Adaptive virtual metrology method based on Just-in-time reference and particle filter for semiconductor manufacturing. Measurement. Volume 168. 15 January 2021.
-
Qibo Yang, Xiang Li, Haoshu Cai, Yuan-Ming Hsu, Jay Lee, Chun Hung Yang, Zong Li Li, Ming Yi Lin. Fault prognosis of industrial robots in dynamic working regimes: find degradation in variations. Measurement. 2020.
-
Elisa Negri, Vibhor Pandhare, LauraCattaneo, Jaskaran Singh, Marco Macchi, Jay Lee. Field-synchronized Digital Twinframework for production scheduling with uncertainty. Journal of Intelligent Manufacturing. October. 2020.
-
Wenzhe Li, Xiaodong Jia, Xiang Li, Yinglu Wang, Jay Lee. A Markov model for short term wind speed prediction by integrating the wind acceleration information. Renewable energy. 2020. Volume 164. February 2021. Pages 242-253.
-
Cai, Haoshu, Jianshe Feng, Feng Zhu, Qibo Yang, Xiang Li, Jay Lee. Adaptive virtual metrology method based on Just-in-time reference and particle filter for semiconductor manufacturing. Measurement. Volume 168. 15 January 2021.
-
Shahin Siahpour, Xiang Li, Jay Lee. Deep learning-based cross-sensor domain adaptation for fault diagnosis of electro-mechanical actuators. International Journal of Dynamics and Control. 2020.
-
Cai, Haoshu, Jianshe Feng, Wenzhe Li, Yuan-Ming Hsu, Jay Lee. Similarity-based Particle Filter for Remaining Useful Life prediction with enhanced performance. Applied Soft Computing. 2020, 94.
-
Li, P., Jia, X., Sumiya, M., Kamaji, Y., Ishiguro, M., Pahren, L. and Lee, J., A Novel Method for Deposit Accumulation Assessment in Dry Etching Chamber, IEEE Transactions on Semiconductor Manufacturing, 32(2), pp.183-189, 2019.
-
Cai, H., Jia, X., Feng, J., Yang, Q., Hsu, Y.M., Chen, Y. and Lee, J., A Combined Filtering Strategy for Short Term and Long-Term Wind speed Prediction with Improved Accuracy, Journal of Renewable energy, 136, pp.1082-1090, 2019.
-
Li, F., Ren, G. and Lee, J., Multi-step Wind Speed Prediction based on Turbulence Intensity and Hybrid Deep Neural Networks, Journal of Energy Conversion and Management, 186, pp.306-322, 2019.
-
Lee, J., Singh, J. and Azamfar, M., “Industrial AI: is it manufacturing’s guiding light?” Journal of Manufacturing Leadership 2019:26–36, 2019.
-
Lee, J., Azamfar, M. and Singh, J., A Blockchain enabled Cyber-Physical System Architecture for Industry 4.0 Manufacturing Systems, Manufacturing Letters, 20, pp.34-39, 2019.
-
Pan, Y., Hong, R., Chen, J., Singh, J. and Jia, X., Performance Degradation Assessment of a Wind Turbine Gearbox based on Multi-sensor Data Fusion, Mechanism and Machine Theory, 137, pp.509-526, 2019.
-
Feng, J., Jia, X., Zhu, F., Iskandar, J, Lee, J., An Online Virtual Metrology Model with Sample Selection for Tracking of Dynamic Manufacturing Processes with Slow Drift, IEEE Transactions on Semiconductor Manufacturing, Vol. 32, No. 4, Nov. 2019.
-
Li, F., Ren, G., Lee, J., Multi-step Wind Speed Prediction based on Turbulence Intensity and Hybrid Deep Neural Networks, Journal of Energy Conversion and Management, Vol. 185, pp.306-322, 2019.
-
Feng, J., Jia, X., Zhu, J., Yang, Q., Y., Pan, and Lee, J., An Intelligent System for Offshore Wind Farm Maintenance Scheduling Optimization Considering Turbine Production Loss, Journal of Intelligent & Fuzzy Systems, Vol. 37, pp.6911-6923, 2019.
-
Shi, Z., Liu, Z., & Lee, J., An Auto-associative Residual-based Approach for Railway Point System Fault Detection and Diagnosis, Measurement, 119, 246-258, 2018.
-
Hossein Davari Ardakani, and Lee. J., "A Minimal-Sensing Framework for Monitoring Multistage Manufacturing Processes Using Product Quality Measurements," Machines 6.1, 2018.
-
Di, Y., Jin, C., Bagheri, B., Shi, Z., Ardakani, H. D., Tang, Z., & Lee, J., Fault Prediction of Power Electronics Modules and Systems Under Complex Working Conditions, Computers in Industry, 97, 1-9, 2018.
-
Zhao, M., Jia, X., Lin, J., Lei, Y., & Lee, J., Instantaneous Speed Jitter Detection via Encoder Signal and its Application for the Diagnosis of Planetary Gearbox, Journal of Mechanical Systems and Signal Processing, 98, 16-31, 2018.
-
Li, Pin, Jia, X, Feng, J.S., Davari, H., Qiao, G., Hwang Y.C., Lee, J., Prognosability Study of Ball Screw Degradation Using Systematic Methodology, Journal of Mechanical Systems and Signal Processing, Feb 2018.
-
Jia, X., Zhao, M., Di, Y., Yang, Q., & Lee, J., Assessment of Data Suitability for Machine Prognosis using Maximum Mean Discrepancy, IEEE Transactions on Industrial Electronics, 65(7), 5872-5881, 2018.
-
Jia, X., Di, Y., Feng, J., Yang, Q., Dai, H., and Lee, J., "Adaptive Virtual Metrology for Semiconductor Chemical Mechanical Planarization Process using GMDH-type Polynomial Neural Networks," Journal of Process Control, vol. 62, pp. 44-54, 2018.
-
Jia, X., Zhao, M., Di, Y., Li, P., and Lee, J., "Sparse Filtering with the Generalized lp/lq Norm and its Applications to the Condition monitoring of Rotating Machinery," Mechanical Systems and Signal Processing, vol. 102, pp. 198-213, 2018.
-
Jia, X., Jin, C., Buzza, M., Di, Y., Siegel, D., and Lee, J., "A Deviation Based Assessment Methodology for Multiple Machine Health Patterns Classification and Fault Detection," Mechanical Systems and Signal Processing, vol. 99, pp. 244-261, 2018.
-
Jia, X., Zhao, M., Di, Y., Yang, Q., and Lee, J., "Assessment of Data Suitability for Machine Prognosis Using Maximum Mean Discrepancy," IEEE Transactions on Industrial Electronics, 2018.
-
Lee, J., Davari, H., Singh, J. and Pandhare, V., Industrial Artificial Intelligence for industry 4.0-based Manufacturing Systems, Manufacturing letters, 18, pp.20-23, 2018.
Selected Conference Papers (for the past five years)
-
Han, Xu, Xiaodong Jia, Dai-Yan Ji, and Jay Lee. "Designing Robust Topological Features for Wafer Map Pattern Classification." In 2023 34th Annual SEMI Advanced Semiconductor Manufacturing Conference (ASMC), pp. 1-6. IEEE, 2023.
-
Takanobu Minami, Alexander Suer, Pradeep Kundu, Shahin Siahpour and Jay Lee, “Novel Ensemble Domain Adaptation Methodology for Enhanced Multi-class Fault Diagnosis of Highly-Connected Fleet of Assets, International Conference on Prognostics and Health Management Asian Pacific, Tokyo, Japan, Sept. 11-14, 2023.
-
Pahren, L., Lee, J., Data Augmentation for Quality Inspection in Product Manufacturing, submitted to IFAC AMEST 2022.
-
Taco, J., Gore, P., Minami, T., Kundu, P., Suer, A., Lee, J., A Novel Methodology for Health Assessment in Printed Circuit Boards. PHM Society European Conference, 7(1), 566–562, 2022.
-
Hsu, YM, Lee, J., Novel Wafer Map Image Analysis using Virtual Metrology, ASME MSEC, under review, 2022.
-
John Taco, Brandon Foreman, Pradeep Kundu, and Jay Lee, “A Novel Technique for Data Quality Evaluation in Human Health PHM,” PHM Conference, under review, 2022.
-
Jia, X., Li, W., Wang, W., W., Li, X., Lee, J., Development of Multivariate Failure Threshold with Quantifiable Operation Risks in Machine Prognostics, Annual Conference of the PHM Society 2020.
-
Abhijeet Ainapure, Xiang Li, Jaskaran Singh, Qibo Yang and Jay Lee. Deep Learning-Based Cross-Machine Health Identification Method for Vacuum Pumps with Domain Adaptation. 48th SME North American Manufacturing Research Conference. June 2020.
-
Yinglu Wang, Xiaodong Jia, Xiang Li, Shaojie Yang, Haodong Zhao and Jay Lee. A Machine Vision Based Monitoring System for the LCD Panel Cutting Wheel Degradation. 48th SME North American Manufacturing Research Conference. June 2020.
-
Yang, Q., Singh, J., & Lee, J. Isolation-Based Feature Selection for Unsupervised Outlier Detection. Proceedings of the Annual Conference of the PHM Society. 2019, 10, 1.
-
Xiaodong Jia, Haoshu Cai, Yuanming Hsu, Wenzhe Li, Jianshe Feng, Jay Lee. A Novel Similarity-based Method for Remaining Useful Life Prediction Using Kernel Two Sample Test. Proceedings of the Annual Conference of the PHM Society. 2019, 10, 1.
-
Negri, E., Davari, H.,Cattaneo, L.,Singh, J., Macchi, M., Lee, J., A Digital Twin-based scheduling framework including Equipment Health Index and Genetic Algorithms. 13th IFAC Workshop on Intelligent Manufacturing Systems Oshawa, Ontario, Canada, 12-14 August 2019.
-
Azamfar, M., Jia, X., Pandhare, V.,Davari, H.,Singh, J., Lee, J, 2019. Detection and diagnosis of bottle capping failures based on motor current. 47th SME North American Manufacturing Research Conference, NAMRC 47, Pennsylvania, USA.
-
Pandhare, V., Singh, J. and Lee, J., 2019, May. Convolutional Neural Network Based Rolling-Element Bearing Fault Diagnosis for Naturally Occurring and Progressing Defects Using Time-Frequency Domain Features. In 2019 Prognostics and System Health Management Conference (PHM-Paris) (pp. 320-326). IEEE.
-
X. Jia, C J. Lee, Z Zhang, P. Radecki, J Lee. A Methodology for the early diagnosis of vehicle torque converter clutch degradation. 2019 IEEE International Conference on Automation Science and Engineering. IEEE, 2019.
-
Jianshe Feng, Methodology of adaptive prognostics and health management using streaming data in big data environment. 2019 PHM Society Doctoral Consortium, Sept 24-26, 2019, Tempe AZ, US.
-
Jianshe Feng, Xinyu Du, Mutasim Salman, Wheel bearing fault isolation and prognosis using acoustic based approach. Annual Conference of the Prognostics and Health Management 2019, Sept 24-26, 2019, Tempe AZ, US.
-
Jianshe Feng, Xiaodong Jia, James Moyne, Jimmy Iskandar, Heng Hao, Kommisetti Subrahmanyam, Michael Armacost, Andrei Korobkov, Jay Lee, Pattern-based trace segmentation and feature extraction for semiconductor manufacturing and application to fault detection. Advanced Process Control 30th, Oct 8-11, 2018, Austin, TX, US.
-
Jia, X., Huang, B., Feng, J., Cai, H. and Lee, J., 2018, September. A Review of PHM Data Competitions from 2008 to 2017. In Proceedings of the Annual Conference of the PHM Society (Vol. 10, No. 1).
-
-
Forty years of MEMS research at the Hilton Head Workshop
UMD Professor Reza Ghodssi is president of Transducer Research Foundation (TRF), the organization that sponsors the event.UMD Hosts Industrial AI Forum
High-profile event highlights university’s leadership role in the field.NAMRI Honors Jay Lee With Outstanding Paper Award
UMD professor’s work could help spur use of blockchain in manufacturing.UMD ME Faculty Ranked in Top 2% of Scientists Worldwide
The department is home to some of the most widely-cited researchers in their fields.UMD to Become Hub for Industrial AI
Renowned expert Jay Lee establishes center at Maryland Engineering.Doyenne Reliability Engineering Program
A fellowship for Mozambican women to pursue graduate education in Reliability Engineering at UMDGiving Spotlight: Maria Korsnick
Alumna Maria Korsnick (B.S. nuclear engineering ’86) contributes to the Marvin Roush Fellowship in Risk and Reliability.Clark School Welcomes New Faculty
Ten new faculty members join the Clark School for the 2013-2014 school year.UMD Scientists and Engineers Present Papers at American Chemical Society Meeting
Exploring “the chemistry of energy and food” … and moreClark School Celebrates Hard-Working Staff
At annual event, Dean Pines cites long-serving and new staff members.Jaydev Desai wins seed grant for diagnostic robotic system
Research will aid in prevention and treatment of atrial fibrillation.Other professional society fellows
- American Society of Mechanical Engineers (ASME), Society of Manufacturing Engineers (SME), Prognostics and Health Management (PHM) Society, International Society of Engineering Asset Management (ISEAM)